views
AI and Big Data in Scientific Research: Transforming the Future
Introduction: A Data-Driven Revolution in Science
The scientific world is undergoing a dramatic transformation, thanks to the explosive growth of artificial intelligence (AI) and big data analytics. No longer confined to theory and traditional experimentation, science today is deeply integrated with data-driven models that can process, predict, and even automate discoveries.
From personalized medicine to astrophysics, AI and big data are helping researchers handle complex problems, scale their work, and reach breakthrough conclusions faster than ever. This article explores the profound role that AI and big data play in modern scientific research and how platforms like AAK Tele-Science are supporting this revolution.
Understanding AI and Big Data in Research
-
Artificial Intelligence (AI) involves the simulation of human intelligence by machines, enabling them to learn, adapt, and make decisions.
-
Big Data refers to extremely large datasets that are too complex for traditional data-processing tools. In research, this often includes genomic data, climate models, real-time experimental data, and more.
Together, they empower researchers to:
-
Discover hidden patterns
-
Predict experimental outcomes
-
Automate repetitive tasks
-
Visualize data at scale
Why AI and Big Data Matter for Researchers
Scientific inquiry traditionally relied on observation, hypothesis, and experimentation. While these fundamentals still apply, the tools have changed:
-
Volume: Research data is now being generated at an exponential rate.
-
Velocity: Real-time data processing is crucial for applications like weather forecasting or financial modeling.
-
Variety: Researchers deal with structured, unstructured, textual, and visual data.
-
Veracity: Ensuring data integrity is vital in sensitive fields like medical research.
This is where AI steps in to support interpretation and insight extraction.
Real-World Applications in Scientific Domains
1. Healthcare and Genomics
AI models can analyze large genomic datasets to predict disease susceptibility and identify drug targets. Machine learning also helps personalize treatment plans by analyzing patient histories, genetic makeup, and trial data.
2. Environmental and Climate Science
Big data from satellites, sensors, and climate models is processed using AI to predict natural disasters, track environmental changes, and support sustainable planning.
3. Physics and Astronomy
In astrophysics, AI helps identify patterns in massive sky survey data, such as detecting new planets or classifying galaxies. AI also optimizes data from particle accelerators like CERN.
4. Chemistry and Material Science
AI-driven simulations speed up the discovery of new materials and chemical compounds by predicting molecular behavior under different conditions.
5. Agricultural Research
AI helps in analyzing soil health, predicting crop yields, and monitoring pests using drone imagery and climate models, enabling smarter farming strategies.
How AI Enhances Research Efficiency
• Automated Data Processing
AI algorithms can clean and organize unstructured datasets faster than any human, freeing researchers to focus on high-level tasks.
• Hypothesis Generation
AI can scan scientific literature and databases to suggest potential research directions or combinations that haven't been tried yet.
• Pattern Recognition
AI systems like neural networks excel at recognizing complex patterns—crucial in fields like neurobiology, where brain activity must be mapped and interpreted.
• Predictive Modeling
By analyzing historical data, AI can forecast future outcomes, saving time and resources in experimental design.
Big Data Analytics: The Engine of Modern Science
Big data analytics provides the infrastructure and algorithms to handle vast research datasets. It involves:
-
Data Collection: Sensors, lab equipment, surveys, simulations
-
Data Storage: Cloud-based platforms like AWS, Google Cloud, or Azure
-
Data Processing: Using Hadoop, Spark, or AI frameworks like TensorFlow
-
Visualization: Tools like Tableau or Power BI help researchers see patterns and anomalies
Benefits of Using AI and Big Data in Research
-
🔍 Deeper Insights: Uncover relationships and interactions not visible with traditional analysis.
-
⚙️ Faster Turnaround: Cut weeks or months off the research cycle with automated data processing.
-
📊 Scalability: Analyze global datasets in seconds using scalable cloud computing resources.
-
💡 Innovation Acceleration: AI’s ability to simulate and suggest experiments leads to faster scientific breakthroughs.
Challenges in Adoption
Even with all its advantages, using AI and big data in research isn’t without hurdles:
-
Skill Gap: Many researchers lack expertise in AI programming and data science.
-
Data Privacy: Especially in biomedical research, ethical handling of personal data is a concern.
-
Bias in Models: AI is only as unbiased as the data it's trained on—something that requires careful oversight.
-
Cost of Infrastructure: High-performance computing tools can be expensive, though cloud models are reducing barriers.
AAK Tele-Science: Empowering Data-Driven Research
AAK Tele-Science is bridging the gap between science and advanced digital tools by providing:
-
Cloud-Based Analytics Environments: Ready-to-use platforms for researchers to run machine learning models and process big data sets.
-
AI-Integrated Research Tools: Pre-built modules for data cleaning, predictive modeling, and visualization.
-
Training & Tutorials: Resources to upskill researchers in AI, coding, and data science best practices.
-
Partnerships with AI Labs: Collaborations that bring academic researchers in touch with tech experts to boost research quality.
Future Outlook: AI-Powered Research Ecosystems
In the next decade, we’ll see AI playing an even bigger role in research:
-
Self-Driving Labs: Robotic labs that automatically run and optimize experiments.
-
AI Peer Review: Intelligent systems that scan research for integrity and novelty.
-
Decentralized Data Sharing: Blockchain and federated learning will enable secure sharing of sensitive data for AI training.
With platforms like AAK Tele-Science leading the charge, the future of research looks faster, smarter, and more collaborative.
Conclusion
AI and big data are not just supporting tools—they are becoming the core of how research is conducted in the 21st century. By automating analysis, predicting outcomes, and uncovering new scientific relationships, these technologies are transforming disciplines and democratizing access to innovation.
For researchers and institutions ready to harness this power, AAK Tele-Science provides the digital foundation to explore, collaborate, and lead in the data-driven age of science.
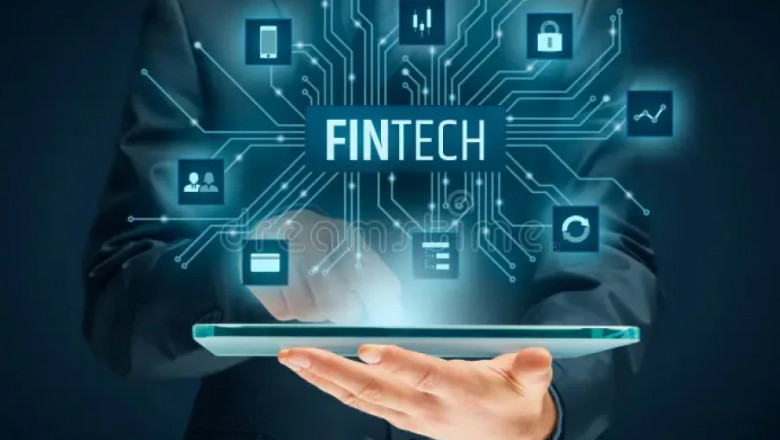

Comments
0 comment